You've collected all your data and you now want to generate a lovely graph... But what does it actually tell you? More importantly, does it tell you what you actually want to know.
More often than not, data collection (particularly wellness) is used as a tool to establish baselines, averages and thresholds. Now, I am a fan of this. However, it is important to understand what the averages mean and how they are being portrayed in your data visualisation.
The problem with averages are that they are exactly that - the average score of all data points in that range. Why is this a problem? The average itself isn't, its the data from which it is calculated. Let's give a working example - if we have 6 players who submit soreness scores (1 =very sore, 5 = not sore at all), it may look like this:
P1 - 3
P2 - 3
P3 - 5
P4 - 5
P5 - 1
P6 - 1
Average = 3
So from this data you may decide that the squad isn't overly sore so training can continue as normal. You may also look at this data a little more closely and decide some players are particularly sore, so split the group in 2, with anyone below a score of 3 training on a reduced load. Now look what happens when Only 3 of your players complete the questionnaire:
P1 - 3
P2 -
P3 - 5
P4 - 5
P5 -
P6 -
Average = 4.3
From this, you may decide that the squad is not sore, so you can push them or even up the training load. What about those who didn't fill it in and are now having increased loading when already sore? Hopefully you can already see how problems can easily start to occur. Now consider when this is spread across greater squad sizes and playing positions.
Real Life Examples
Below are a handful of different graphs displaying wellness data across an entire you. All the visualisations come from the exact same data.
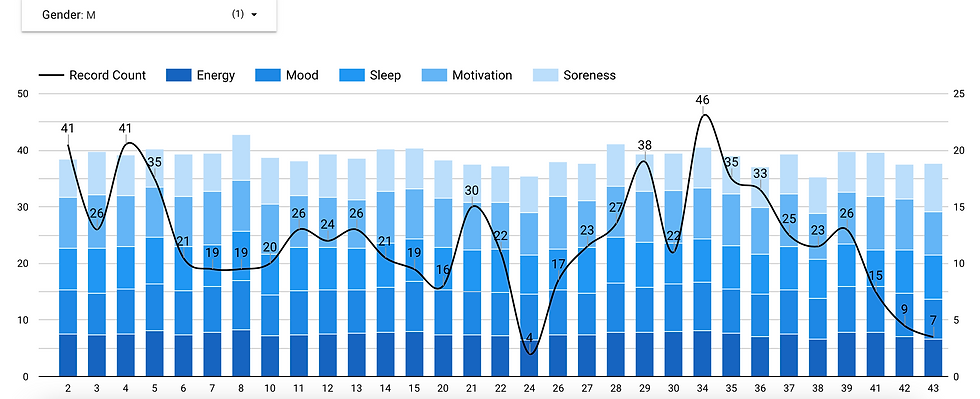
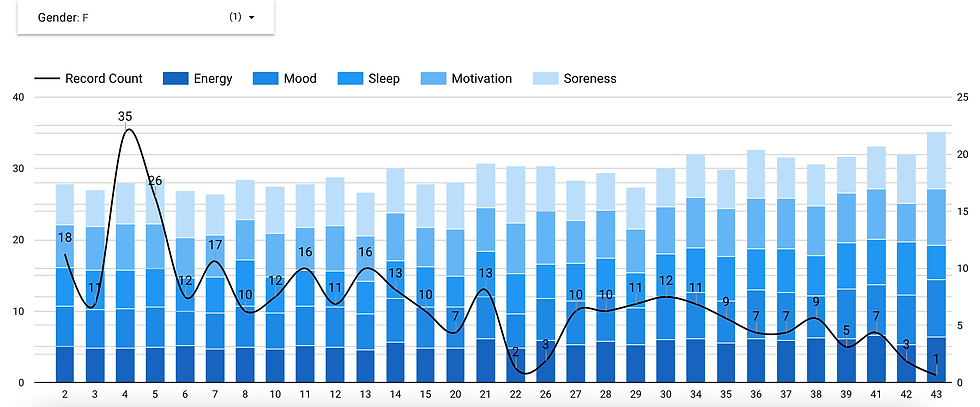
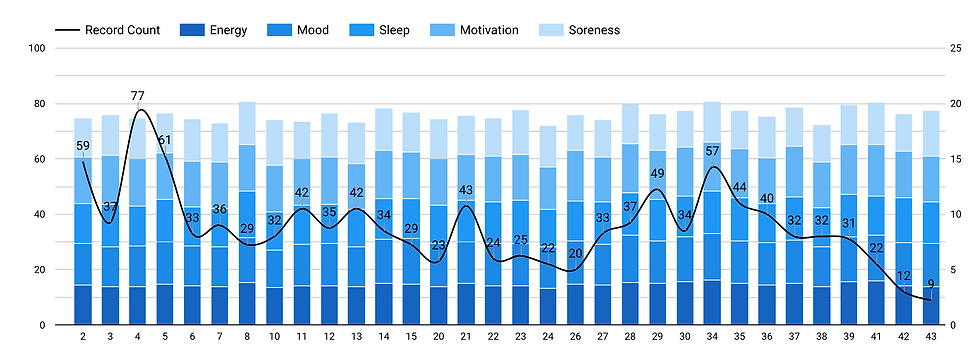
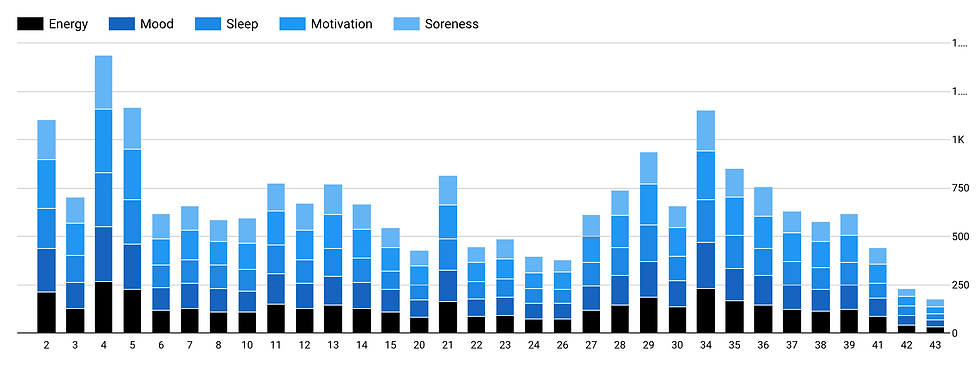
Hopefully, you can see how the problems can increase when we visualise our data. As mentioned before, the graphs above are all from the same data. So, how can thy look so different and what issues might it cause? To help, I should explain what each graph shows.
Graph 1
The average of all male scores + how many questionnaires were filled in.
Graph 2
The average of all female scores + how many questionnaires were filled in.
Graph 3
The average of all male and female scores + how many questionnaires were filled in.
Graph 5
The SUM of all completed questionnaires for all male and female scores.
If you were to take your info from graph 5, you may mistake high numbers of completed questionnaires as high wellness scores and vice versa. If you were to take the data from the combined + total filled in - you have a better understanding, but it can still be very different for each gender.
The Solution
I do feel like I am on repeat a little bit here! The best way to understand your data, is to understand exactly what it is you are looking for when you collect it. From here you can separate it out to give an accurate picture of what you want to know. This may mean digging a little deeper and establishing averages for each squad, playing position, or even each individual. You may also want to come up with some visual system or representation that highlights difference in the amount of data collected.
Once you you know what you're looking for and where to find it, you can decide how to show it. Are you looking for the SUM (everything added together), the average (mid point) or MAX/MIN (highest or lowest data points).
Comentarios